Time Series Forecasting | Autoencoder Echo State Network
Proposing a novel integrated approach, introduced as autoencoder ESN (AE-ESN), for long-term prediction of highly nonlinear time series. This architectur demonstrates huge improvements in the predcition accuracy and robustness.
Echo state networks (ESNs) provide a computationally efficient approach for multi-step-ahead prediction of complex time series, especially for highly nonlinear or chaotic cases, where the prediction superiority of commonly used deep-learning techniques does not carry over. However, due to the random nature of ESNs and the intrinsic sensitivity of such networks to the hyperparameters and the values of the untrained network parameters, finding an appropriate set of values is a challenging step in constructing such networks. Consequently, despite their efficiency, ESNs are rarely considered a practical forecasting technique in real-world applications. Building on recent results in long-term prediction of complex nonlinear time series, this work introduces and evaluates an integrated architecture in which a long short-term memory (LSTM) autoencoder is integrated into the ESN framework. In this approach, the autoencoder learns a compressed representation of the input nonlinear time series. Then, the trained encoder serves as a feature extraction component feeding the learned features into the recurrent reservoir in the echo state network (ESN). The proposed approach is evaluated using synthetic and real-world experimental complex time series representing voltage recordings from cardiac cells, which have been shown to exhibit nonlinear and chaotic behavior. Not only do the computational results demonstrate improvements in prediction accuracy and robustness compared to mainstream data-driven approaches for chaotic time series forecasting, such as the baseline and physics-informed ESNs, but the representation provided by the feature extraction component in this technique also removes the requirement in related previous work for explicitly introducing additional exogenous time series to the network, which normally are not available in real-world applications.
The details and results of this research is published in in
"Chaos: An Interdisciplinary Journal of Nonlinear Science"
by AIP, and selected as theFeature Article 🎖
by the editors.
The full PDF file and the corresponding supplementary material is available to download.
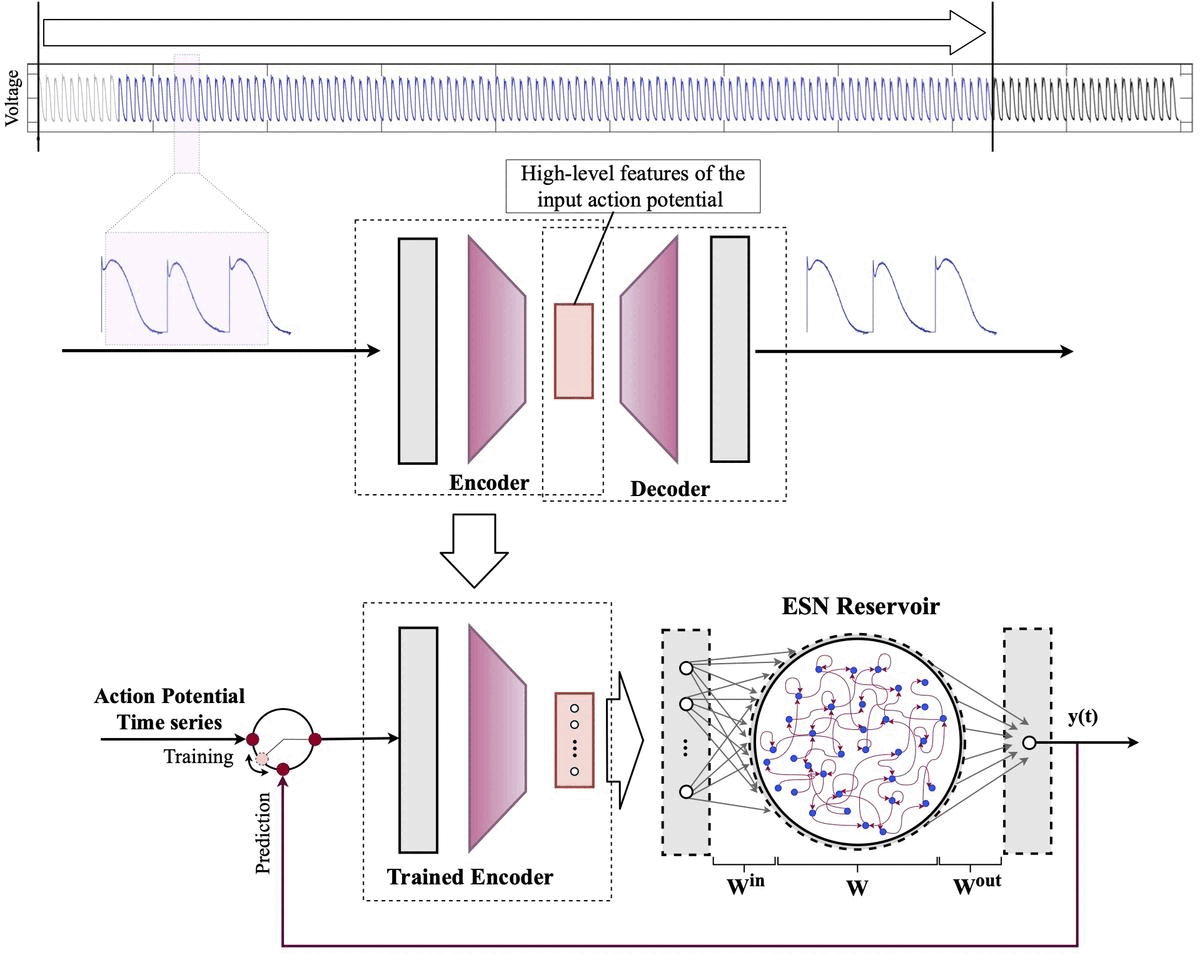
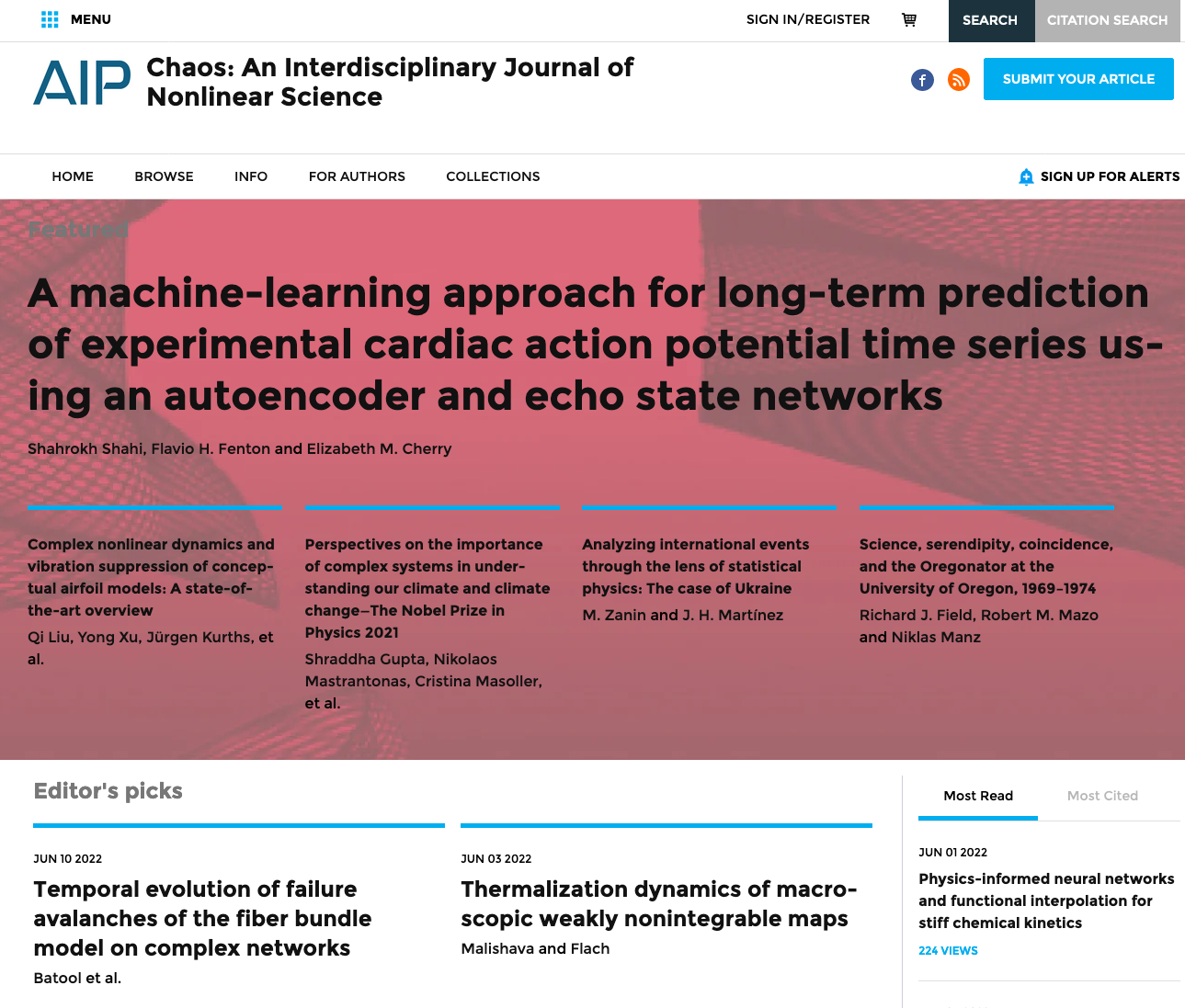