Our New Deep Learning Paper is Published!
Our recent paper, "Prediction of Chaotic Time Series Using Recurrent Neural Networks and Reservoir Computing Techniques: A Comparative Study"
, has been accepted to be published in "Machine Learning with Applications"
published by Elsevier. The full text paper is available in publications section.
Abstract
In recent years, machine-learning techniques, particularly deep learning, have outperformed traditional time-series forecasting approaches in many contexts, including univariate and multivariate predictions. This study aims to investigate the capability of (i) gated recurrent neural networks, including long short-term memory (LSTM) and gated recurrent unit (GRU) networks, (ii) reservoir computing (RC) techniques, such as echo state networks (ESNs) and hybrid physics-informed ESNs, and (iii) the nonlinear vector autoregression (NVAR) approach, which has recently been introduced as the next generation RC, for the prediction of chaotic time series and to compare their performance in terms of accuracy, efficiency, and robustness. We apply the methods to predict time series obtained from two widely used chaotic benchmarks, the Mackey-Glass and Lorenz-63 models, as well as two other chaotic datasets representing a bursting neuron and the dynamics of the El Nino Southern Oscillation, and to one experimental dataset representing a time series of cardiac voltage with complex dynamics. We find that even though gated RNN techniques have been successful in forecasting time series generally, they can fall short in predicting chaotic time series for the methods, datasets, and ranges of hyperparameter values considered here . In contrast, for the chaotic datasets studied, we found that reservoir computing and NVAR techniques are more computationally efficient and offer more promise in long-term prediction of chaotic time series.
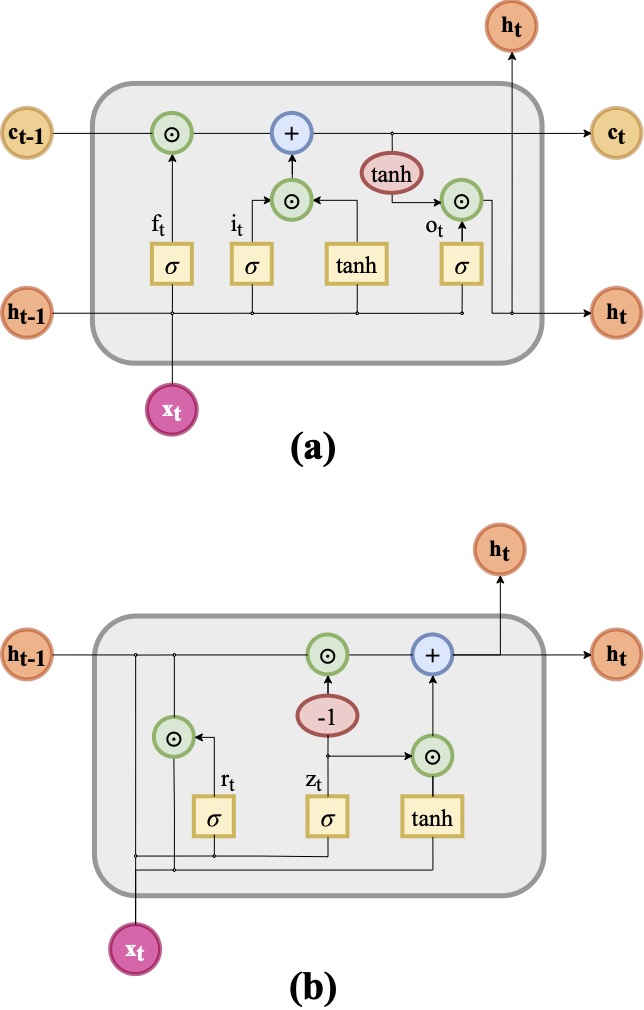
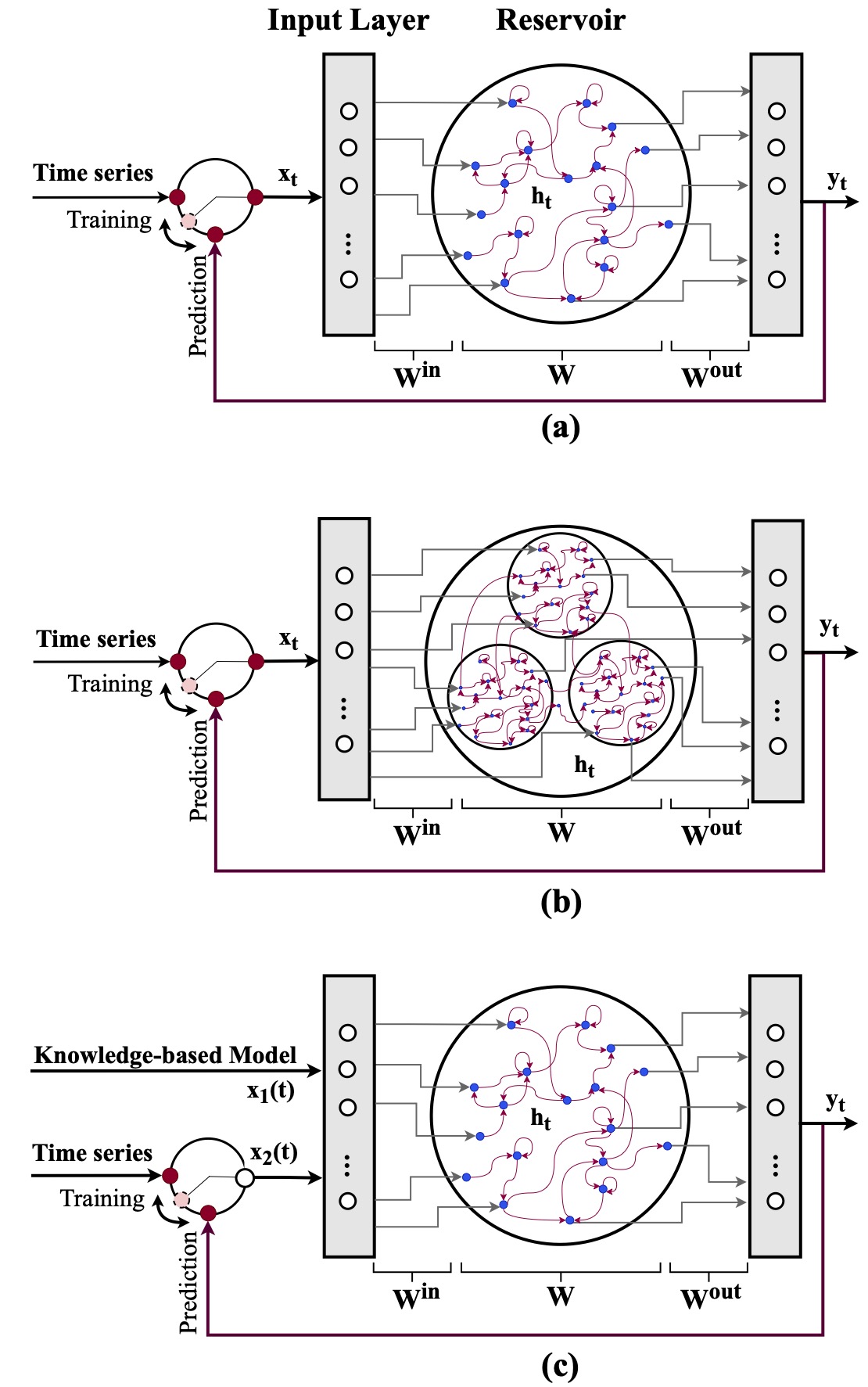
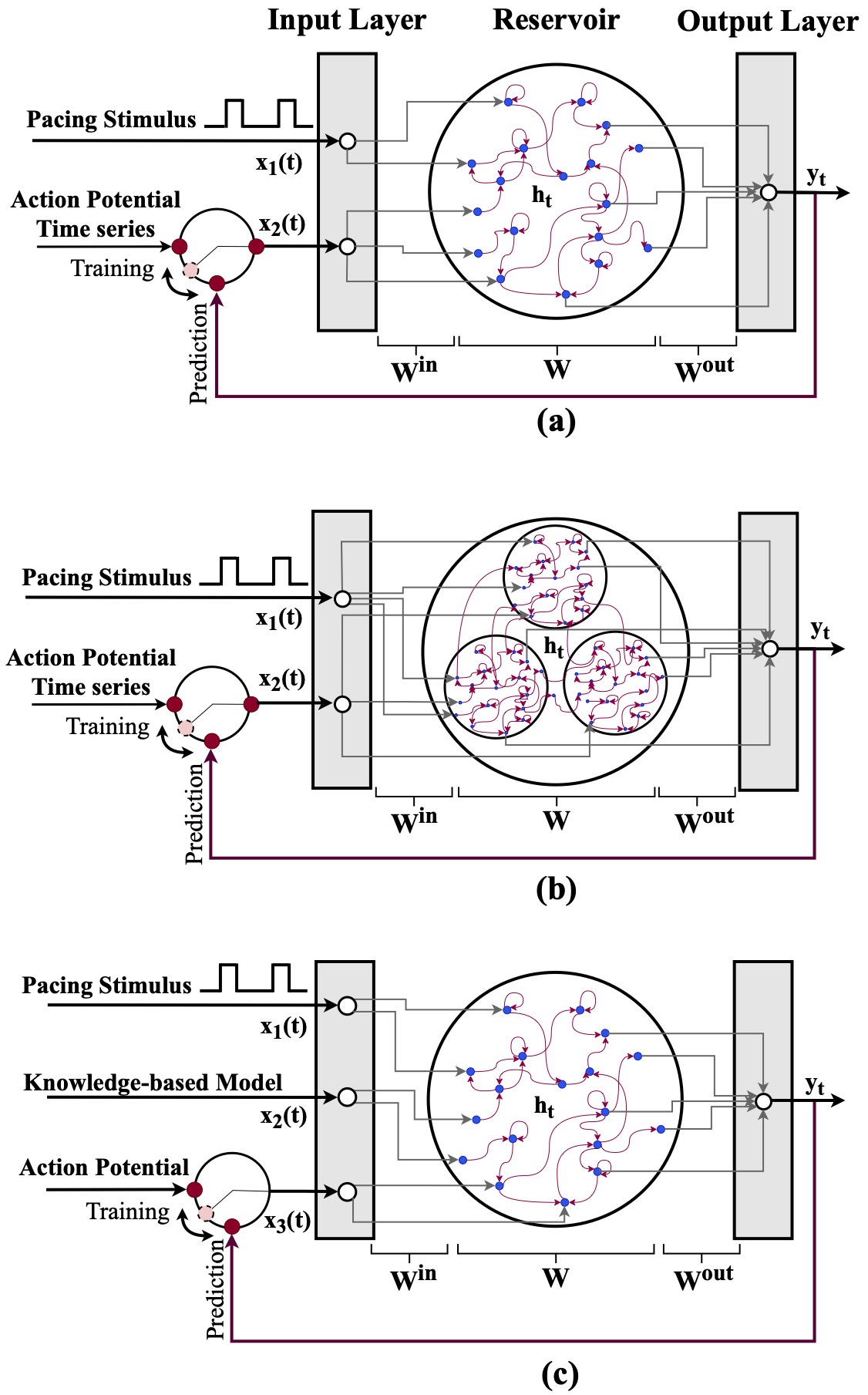
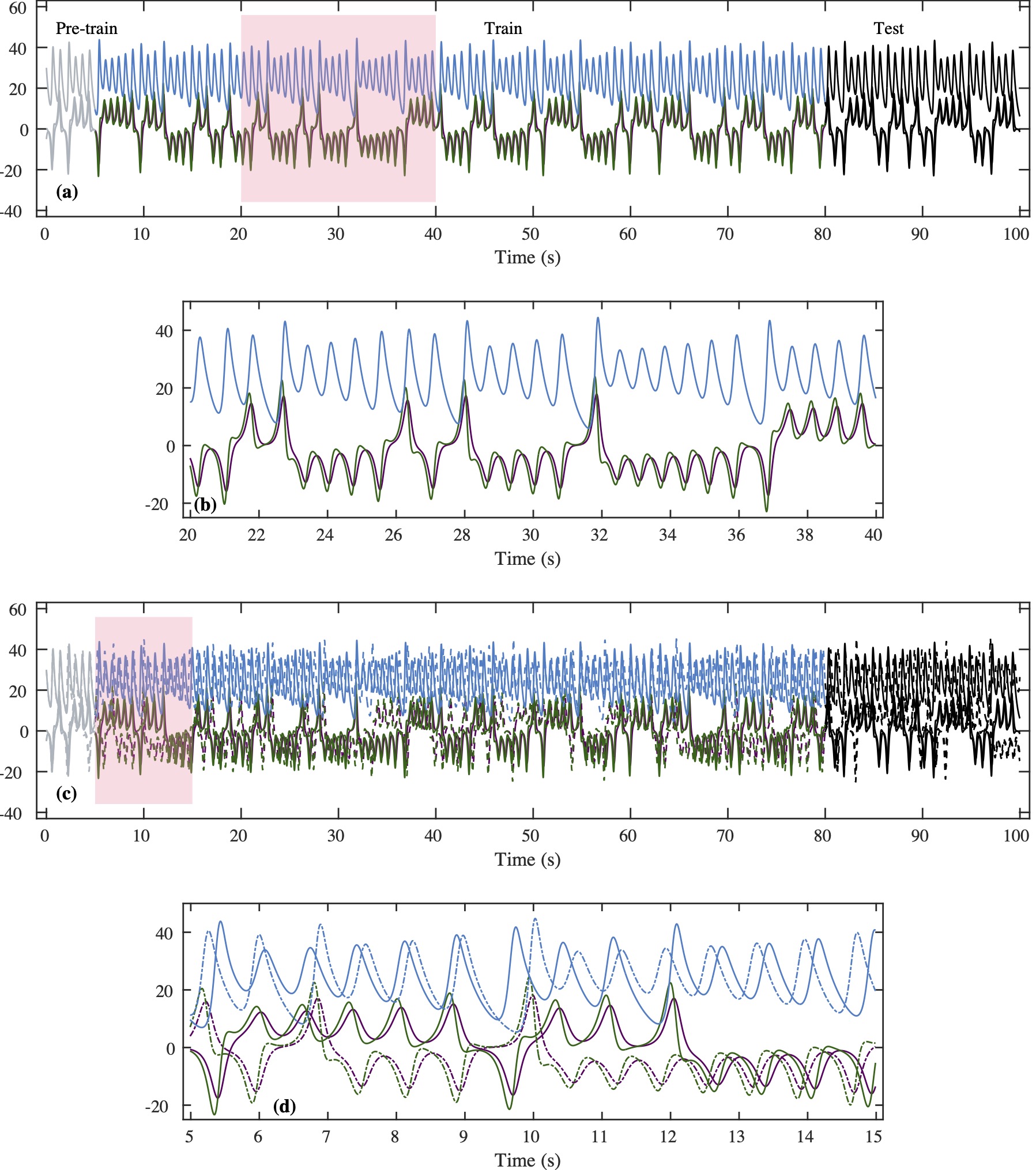
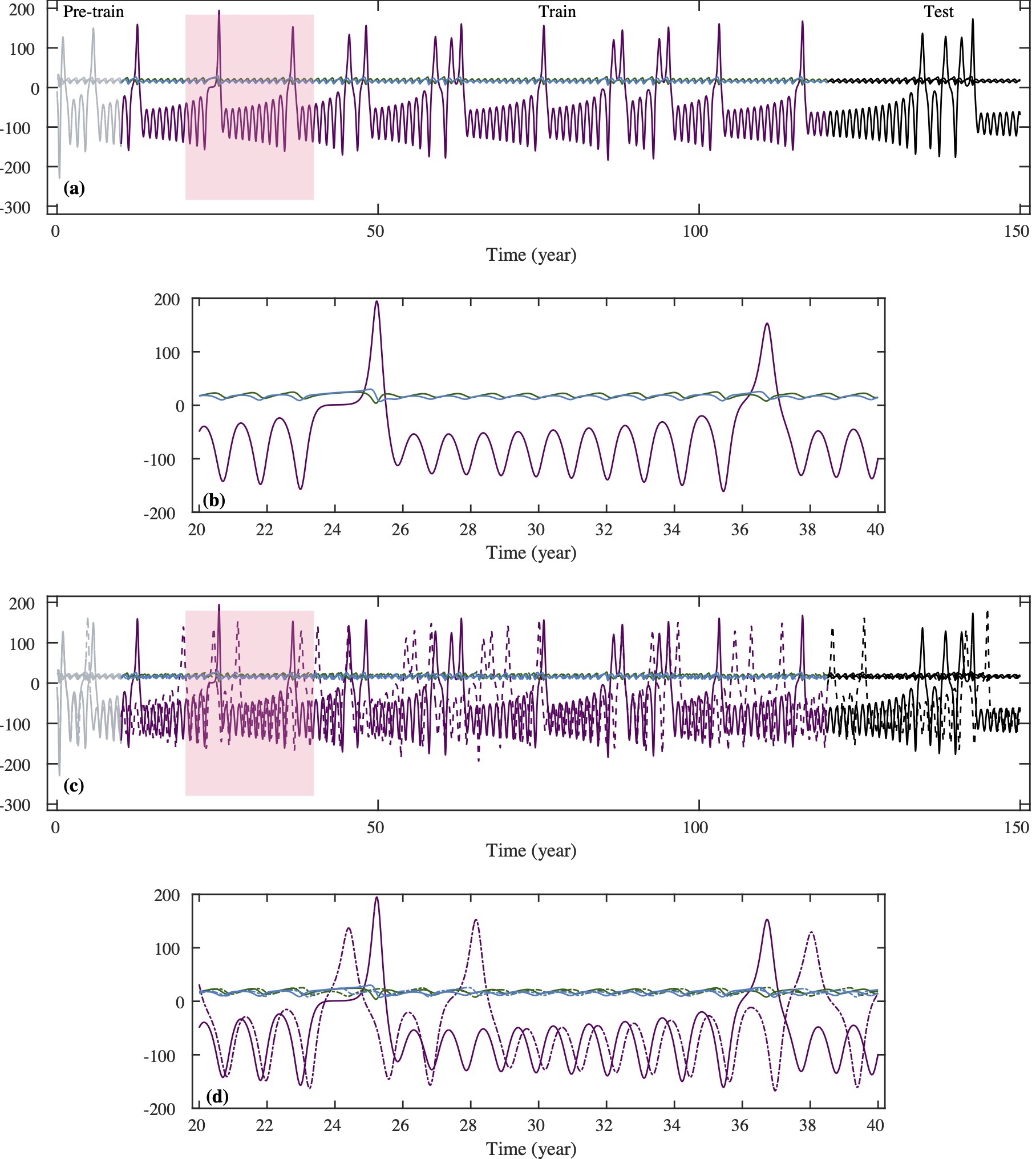
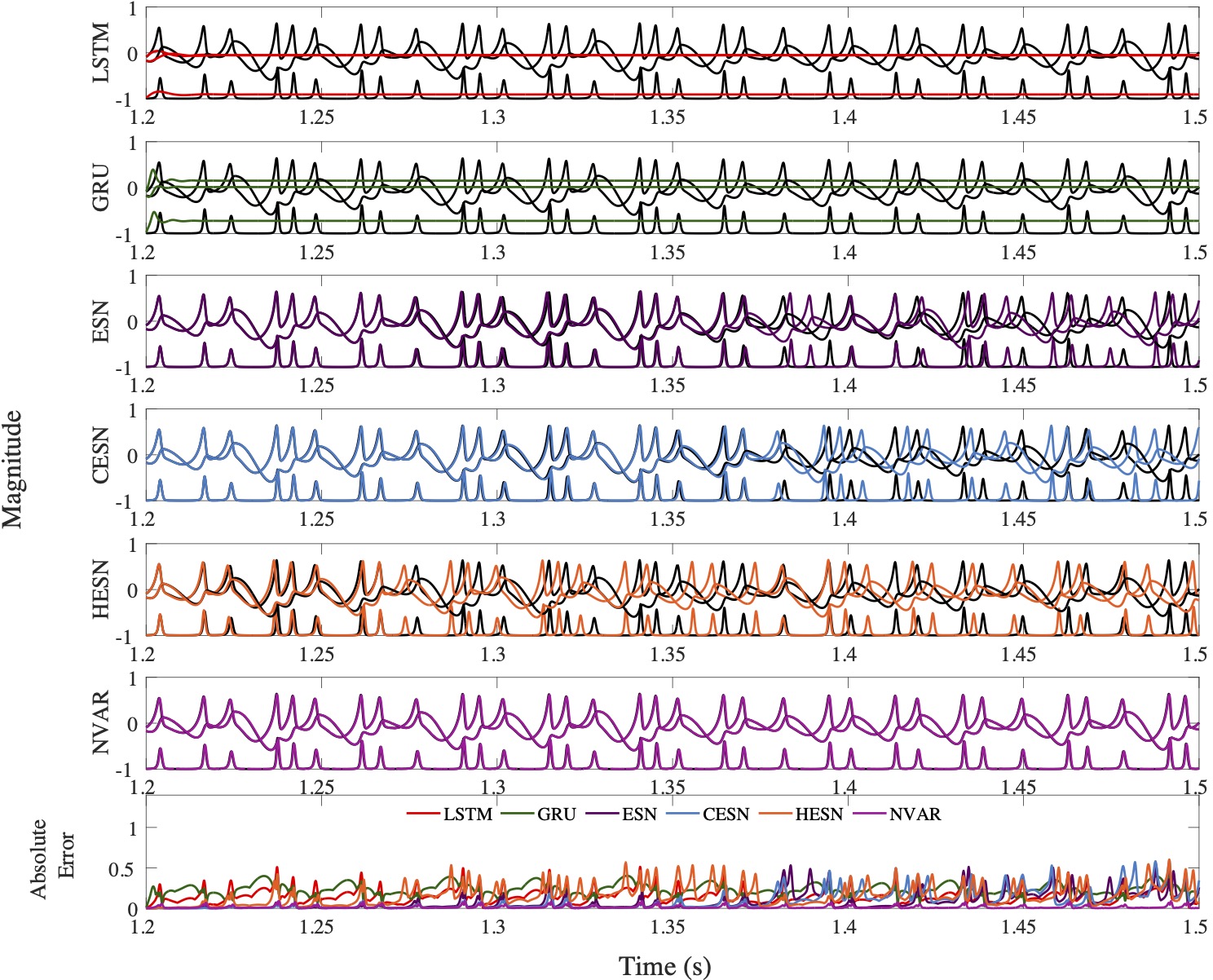
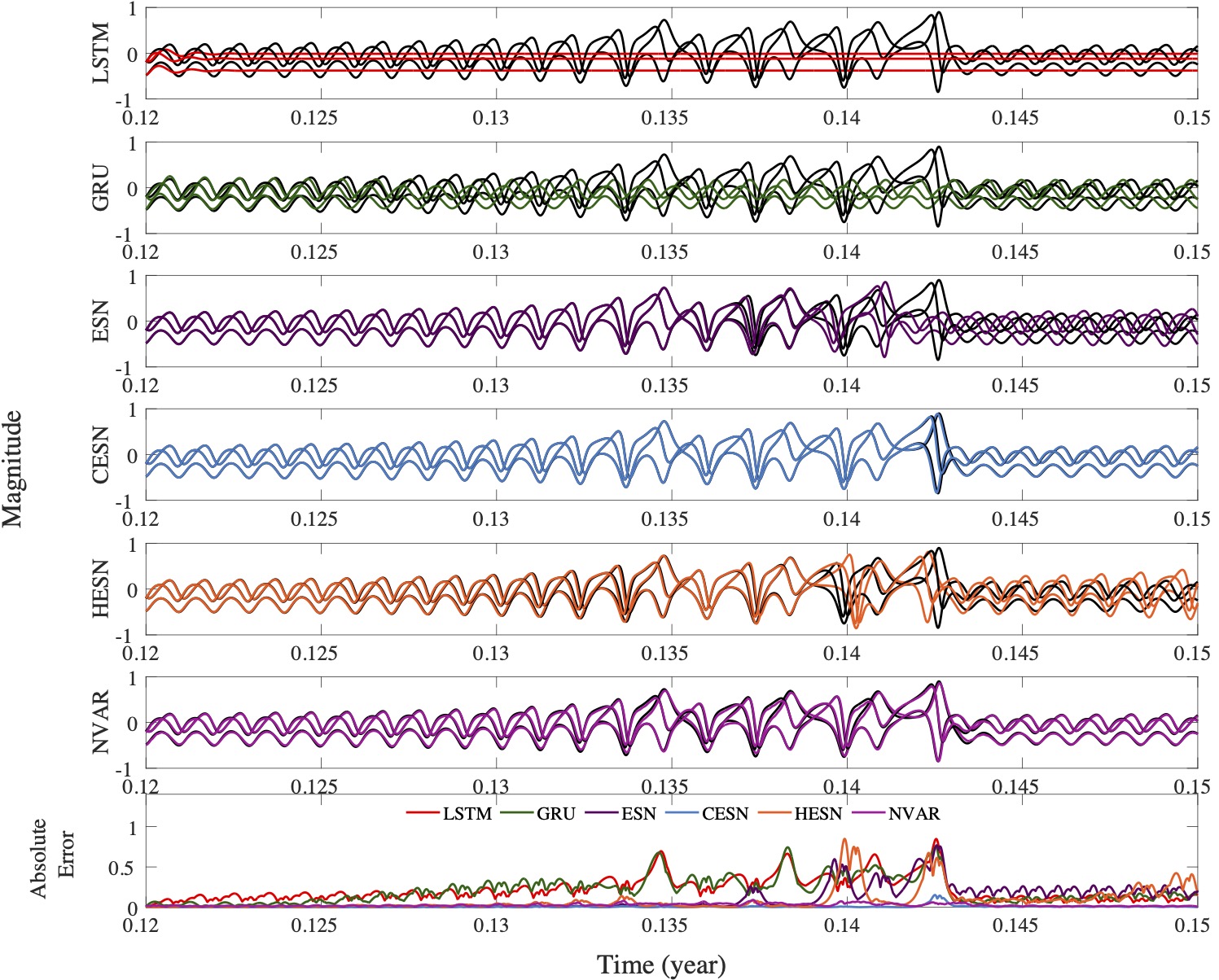